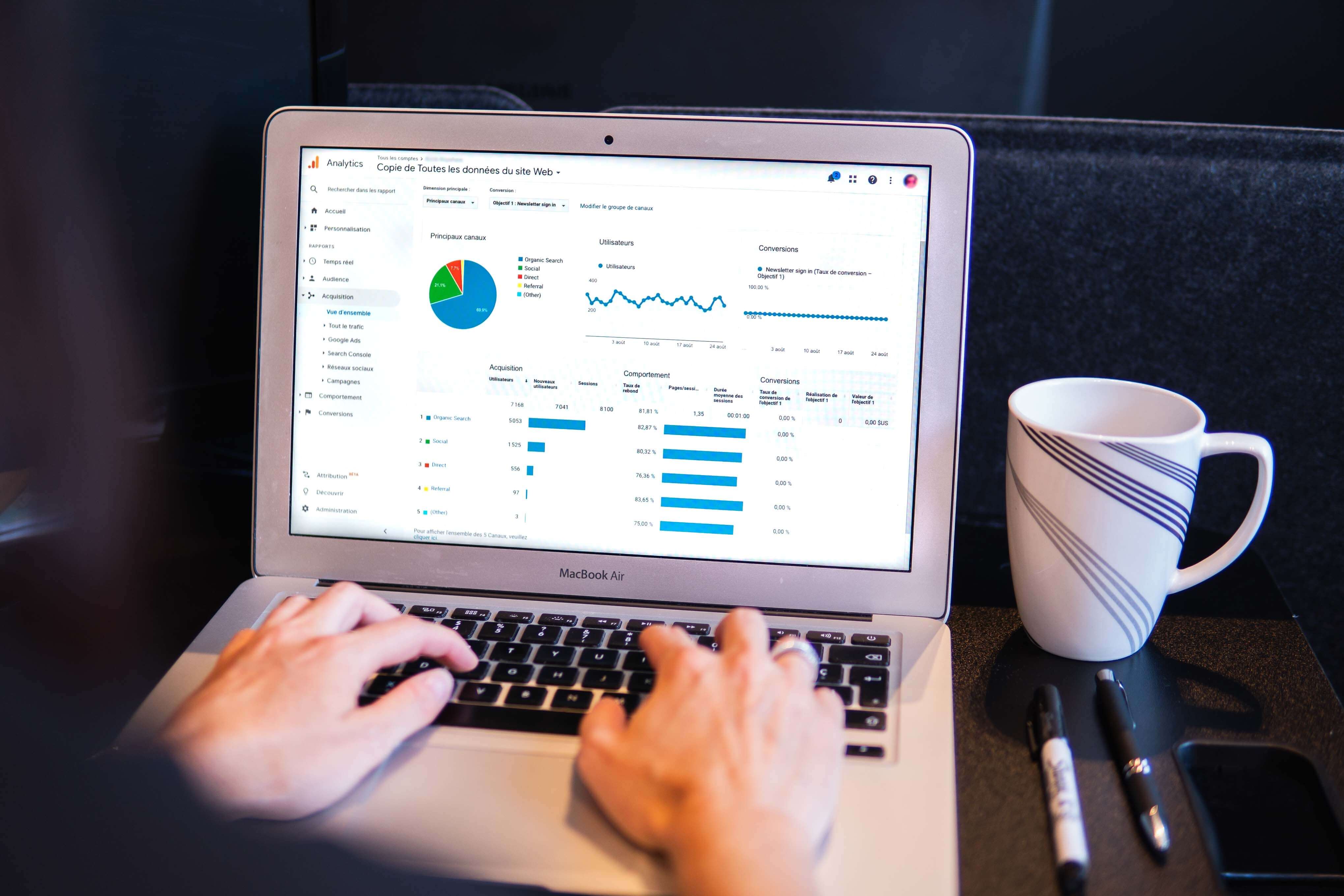
What is predictive analytics?
Predictive analytics is a subset of advanced analytics that uses previous data to create predictions about future events using statistical modelling, data mining techniques, and machine learning. Businesses use data analytics to make data-driven decisions about meaningful opportunities and to limit risks.
Predictive analysis have been used for decades but have recently become increasingly accessible and high-performing, rendering them an invaluable asset for any company looking to level up.
Predictive analytics: overview
Multiple software applications and tools are used for predictive analytics. Generally speaking, these applications use algorithms and methodologies to measure past data. These numbers, which are stored in data warehouses, give insights and forecasts of future behavior for persons, robots, and a variety of other entities. Learn how to successfully incorporate a prediction model into your company’s structure with these 8 keys steps for a successful digital transformation.
Machine learning vs predictive analytics
Business intelligence systems have significantly evolved since their beginnings in the early 1990s. Indeed, every business intelligence tool has progressed to gather data through various methods and procedures. Experts will agree that machine learning is fundamental to predictive analytics. While there are specific differences between both, they have increasingly become used interchangeably.
What are the predictive analytics tools?
Entire school curriculums are geared toward understanding machine learning techniques. Some well-known and widely used predictive analytics tools include classification models, regression models, decision trees, and neural networks.
While the terminology often varies, analytics tools more or less all do the same thing. They gather rich data to illustrate actionable courses of action and insights for businesses. For further information on different types of predictive analytics, read IBM’s report on predictive analytics.
The benefits of predictive analytics
Improving customer loyalty
Our shopping experiences are progressively becoming more personalized. These highly individualized experiences are, in part, due to a prediction model. Predictive analytics are used to understand population trends and behaviors. This, in turn, improves the service and performance of various companies and leads to customer loyalty.
Identify potentially profitable customers
An array of industries uses predictive analytics. Retail companies have been gathering data on consumer behaviors to analyze the effectiveness of various strategies. Metrics such as purchasing time, day and customers’ genders are all stored to plan successful promotional events and future campaigns. These customer insights help companies continually refine their target audience’s needs and thus improve overall customer satisfaction.
Improvement of decision-making
Beyond helping one determine the best course of action, prediction analysis helps improve various operations. Many manufacturers will use machine learning techniques to manage inventory and resources, therefore maximizing efficiency and organization. Predictive analytics help business leaders make better decisions regarding their resources, priorities and planning.
Risk prediction and quantification
Machine learning techniques allow companies to gather past tendencies and behaviors and make enlightened decisions on whether or not they should proceed or work with certain people and entities.
While many sectors use predictive analytics, a well-known example of its usage is for credit scores. A credit score is based on past purchases and behaviors and can provide an accurate indicator of future patterns. Banks use predictive analytics to determine whether or not they will proceed with loans, insurance and various other collaborations.